SUMMARY OF PROCEEDINGS AND RESEARCH RECOMMENDATIONS
BREAKOUT GROUP 1
BIODIVERSITY INDICATORS AND MODELING:
UNDERSTANDING THE
DISTRIBUTION AND CONDITION OF BIODIVERSITY
Co-Chairs:
Donat Agosti, American Museum of Natural History, New York, NY
Debra Moskovits, The Field Museum, Chicago, IL
Yeqiao Wang, University of Illinois, Chicago, IL (Now at the University of Rhode Island, RI)
Closing the loop.
Conservation of biological diversity can be viewed as a feedback loop that includes data collection, data analyses, recommendations for actions, policy decisions, and implementation of appropriate public policy by decision-makers. But one link must be reinforced: the feedback link between policymakers and data collectors. We must close this gap through standardized data sets and appropriate technologies.
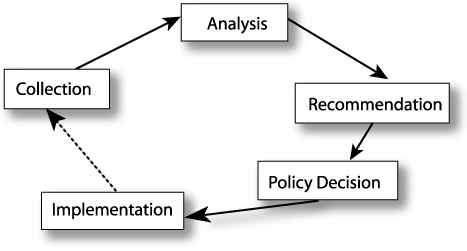
Donat Agosti, of the American Museum of Natural History, cited two examples of cases in which such a feedback loop exists and where data collection plays a crucial role in implementing environmental action: the Intergovernmental Panel on Climate Change and The Montreal Protocol on Substances that Deplete the Ozone Layer. While the 1992 Earth Summit served as a forum for the scientific community and policymakers to discuss ramifications of the loss of biodiversity, the Convention on Biological Diversity placed most emphasis on the action/implementation side of the loop. Only recently has the standardized collection of data been given greater attention. In this regard, innovative technologies will be key in helping us detect and understand the current extent and condition of biological diversity around the world, and the changes in biological diversity over time. Furthermore, a combination of data captured on the ground and through remote sensing will be instrumental in evaluating the effects of implemented conservation policies. These data will be critical in closing the "feedback loop."
To maximize the effectiveness of data collected, Agosti advocated following standard protocols and normalizing the data. A potpourri of data exists; but making the data available in user-friendly databases, and building computing capabilities for storage and retrieval, present serious challenges.
Agosti posed questions that face us today as we set priorities for biological conservation: What is at stake? What elements do we need to study, and what changes do we need to track to be able to make the best possible decisions for conservation? What are the critical scales at which to focus the correct models? These are just a few of the questions this breakout session sought to address.
Museum specimens: spanning the dimensions of time and space.
Biodiversity inventories and monitoring are bringing new life to museum specimens. Such specimens - estimated to number 2.5 billion objects worldwide - represent snapshots of natural history, which, in some cases, go back hundreds of years. Most specimen labels yield point data that indicate where a given species was located and when. These species maps, according to Sasan Saatchi of the California Institute of Technology’s Jet Propulsion Laboratory, can then be coupled with remotely-sensed climate and landscape data for predictive distribution maps.
The interim goal, according to Norman Johnson of Ohio State University, is to provide real-time access, perhaps through Web-based catalogs, to these museum-based data. The bottleneck in the process of providing access is data entry. To derive the spatial and temporal data, the specimen labels must be deciphered. In most cases, the labels include the site of collection, collection date, and other information, such as the collector’s name, collection method, and ecological notes. Label information can be entered into databases at rates ranging from about 10 to 40 specimens per hour in the United States and Canada, in Johnson’s experience.
"Georeferencing" the specimens is the greatest challenge. Over time, place names have changed. Collectors noted locations with various degrees of accuracy. Fortunately, today such variability has largely been eliminated by the advent of global positioning systems with their improved precision and accuracy. Robin Sears of the New York Botanical Garden shed some insight into the extent of the georeferencing problem. She examined museum data on tree species used for timber. Approximately 10% to 30% of the specimens she examined had no coordinate data. The collection sites for 56% of the specimens could only be identified within 10 kilometers.
The group acknowledged that sampling bias is a problem with museum specimens. David Stockwell of the San Diego Super Computer Center pointed out that the random errors that crop up in museum data cancel out while those made in a systematic way do not. Methods do exist to compensate for systematic errors in models. The group acknowledged the need to explore the benefits and limitations of museum data, and to fill the data gaps. Standards are needed for collecting and labeling specimens, as well as measures to evaluate the collecting effort.
Sears, Saatchi, and others in the group reiterated the need for ground-truthing to verify the accuracy of model-predicted species distributions. Sears also pointed out the need for land use and social science data to validate the assumptions we make for models.
To date, publications of monographs have been the only means by which such data exit the museum door and enter the scientific community. Johnson noted that using museum specimens as a source of point data to integrate with environmental data through the application of biodiversity models will provide new avenues of application for museum collections. How, specifically, can these museum specimens be used to monitor and predict trends in biodiversity?
Johnson posited a number of applications. The museum data can suggest indicator species. They can quantitatively identify centers of endemism and indicate species richness. They can predict spread of invasive species. They can aid in selection of biological control agents. These data show us where the species are (or were). By coupling these point data to meteorological data and NASA remote-sensing data, such as vegetation cover and topographic information, we can model the probable distribution of species via predictive models. With such models, we can look for commonalities among models for different species. Such commonalities in turn might serve as surrogate measures of diversity.
Mapping the earth’s biodiversity.
David Stockwell, among others, is building the computer infrastructure for storing and accessing biodiversity data, advancing computing tools for modeling and mapping, and developing a digital library of fauna and flora. Models expand the utility of point data, which are limited because of data scatter and collection bias. If we use the point data in conjunction with remote-sensing data to construct models, we can predict probabilities of species distribution with much better resolution.
Multivariate models can accommodate a wide variety of explanatory variables, including temperature, rainfall, elevation, aspect, slope, relief, geology, potential soil nutrients, and soil wetness. With such models, we can identify potential habitats for a given species. We can also incorporate temporality: by applying the same profile during different seasons, we can predict where the species might migrate. The power of the modeling approach can be harnessed by "layering" species models to create assemblage models, which in turn can be layered to create models of bioregionalization. Stockwell presented a compelling graphical depiction of how model accuracy grows as additional data "layers" are added.
These models are important for reserve system planning and have already been demonstrated in several applications, including:
- Predicting patterns of endemism with 100-meter resolution in New South Wales forests;
- Predicting the potential invasion of Chinese Longhorn Beetles in the United States; and
- Establishing migration routes of South American birds.
Some goals for modeling go beyond presence/absence studies to predicting trends. As Yeqiao Wang of the University of Rhode Island emphasized, dynamic processes - both natural and cultural - drive landscape change. Current models, Wang pointed out, are mostly static approaches. Quantitative landscape modeling requires that time or temporal changes be considered. Effective dynamic mechanisms are demanded to integrate fully physical, biological, and human factors into landscape simulation. Only rarely have socioeconomic, demographic, and driving-factor data been linked with remote sensing data in simulation models. Nevertheless, these ever-changing human factors, especially suburban sprawl, are the main drivers behind changing landscapes and threats to natural ecosystems, according to Wang.
Bridging the gap between conservation biology and global monitoring.
Stockwell enumerated a number of challenges facing biodiversity modelers as they apply NASA’s remote sensing data:
- Which remote sensing data are best for predicting distributions of which taxa?
- How do we incorporate time-varying, remote sensing data into habitat models?
- How do we provide an infrastructure for archiving, accessing, and analyzing remote sensing data?
These all point to ways in which we can close the gap between in situ data and remote sensing data. Wang pointed out how the two types of data complement each other in a spectrum of applications. For example, for assessing fragmentation, remote sensing is good. For assessing degradation, transect data and field data must be incorporated. Data collection in the field is the means by which species composition is determined. Impact analyses are even more complex; here we need data on socioeconomic attributes and drivers, as well as policy analysis. For modeling dynamic mechanisms, data are needed to define interrelationships among ecosystem components.
Saatchi observed that a wealth of new remotely-sensed data (e.g., rainfall, cloud cover, soil types) will be available in the near future. These data will enhance or replace information currently used in models predicting species distributions. For example, improved data on land cover (representing actual rather than predicted cover) will allow us to assess more accurately the current state and distribution of biological diversity, which in turn will provide critical baseline data for future comparisons.
The issue of scale - the optimal spatial resolution at which a particular phenomenon can be observed - must be addressed. No doubt, increased spatial resolution of remote sensing data is a great boon to conservation biologists. But, we must not "lose sight of the forest for the trees" when identifying indicators that cross spatial scales. Wang used an example of how high spatial resolution could lead to false assumptions about a landscape patch. A landscape ecologist on the ground views a patch of grassland with scattered trees in well-spaced clusters, and designates it a savanna. But, remote sensing data might give a much different picture depending upon the resolution. With 30-m Landsat Thematic Mapper data, a one-pixel focus might reveal a grassy spot, leading to the designation of "prairie;" or a partial canopy/partial grassland spot might lead to a "woodland" designation. Digital Multispectral Videography (DMSV) data, with a 2-m resolution can provoke further uncertainty. A one-pixel focus could reveal forest, woodland, or prairie. Transects must be integrated into ecological concepts. We cannot simply apply a model to an entire region based on a limited examination of remote sensing data.
Wang made a number of recommendations based upon his experience with the application of remote sensing data in biodiversity studies and mapping:
- Explore the potential of remote sensing technology for biodiversity conservation.
- Identify biodiversity indicators that can cross spatial scales.
- Develop quantitative models that bridge knowledge from conservation biology and data from remote sensing.
- Integrate data from multiple sources to develop more accurate models.
- Develop dynamic models that incorporate landscape changes and trend studies.
- Develop modeling mechanisms that incorporate drivers to simulate human impacts on natural ecosystems.
The group also developed a "wish list" of remote sensing data that would aid biological conservation efforts. Included on the list were 500-m spatial resolution rainfall data, vegetation seasonality data (daily, monthly, yearly), technologies to detect roads and other human infrastructure under the canopy, "updateable" road maps, locations of dams, availability of radar images, more detailed topographic data, soil and soil-moisture data, geology, and fractional vegetation by pixel.
Looking ahead.
How can conservation biologists best use NASA’s satellite imagery? The group created a list of eight recommendations to further conservation goals through the use of remote sensing data:
- Develop (1) process models integrating spatial and point data, and (2) predictive (forecast) models of the distribution of species, species assemblages, and habitat types.
- Ground models in urgent conservation needs and priority research questions.
- Use the models
- to understand the primary factors (and processes) that drive the distribution of species and species assemblages (to what extent can remotely sensed data identify/quantify distribution of biodiversity? How can point data be extrapolated reliably with the use of remote sensing?);
- to identify centers of endemism; distribution of biodiversity;
- to identify and predict threats to biodiversity (including potential distribution ranges for introduced or invasive species);
- to monitor and predict trends in the distribution of species and species assemblages in reaction to environmental changes (e.g., climate change, fire or suppression of fire) and human impacts (roads, urban sprawl); superimpose maps of diversity and threats and highlight regions of high overlap; and
- to understand the dynamics of habitat patches and the viability of fragmented communities (protected and unprotected) in changing environments.
- Use an iterative approach to modeling biodiversity; emphasize feedback between modeling and ground-truthing to improve accuracy of models; combine remote sensing data and field data.
- Investigate how remote sensing data give us the temporal and spatial context and how they can improve the accuracy of models (predictive and process).
- Make models available and accessible to biologists/conservationists worldwide.
- Identify useful scales for data capture (devise tests to arrive at correct scale).
- Develop sets of indicators that detect change.
- Research what are the key structural indicators that help explain the distribution of biodiversity (species, species assemblages, natural communities).
- Identify indicators of change that can cross spatial scales.
- Identify how NASA captures these "indicators." Specifically, the conservation biology community needs remote sensing data (e.g., Lidar/radar imagery for detecting forest structure, marine community structure, wetlands; biophysical and climatic parameters; and human infrastructure) to integrate with in situ data (field, museum data).
- Develop monitoring designs that use these indicators of change.
- Collect data on a variety of scales, from individually-protected sites to regions and landscapes.
- Standardize primary data acquisition.
- Identify and address existing biases in museum and field data.
- Standardize collection of field data using georeferenced point data.
- Train field-based scientists to use these standard protocols consistently.
- Upgrade systems in museums (and conservation nongovernmental organizations (NGOs)) to make data available.
- Address bottlenecks in the data entry process.
- Develop methods for data archiving.
- Set priorities for data capture in museums and NGOs (into electronic databases), based on key conservation and research needs.
- Use NASA data specifically for conservation purposes (protection, management, restoration, monitoring).
- Obtain more specific data from NASA, if possible.
- A wish list of data requirements includes: 500-m spatial resolution rainfall data; detection of road infrastructure under canopy; "updateable" road maps; locations of dams; Lidar/radar imagery to detect forest structure, wetlands, marine community structure; fractional vegetation by pixel.
Field study is more than a means to verify models. Field data are needed to close the feedback loop. It is the responsibility of conservation biologists to provide this feedback to NASA and policymakers so that data collection - whether from the field or from satellites - can be refined to improve conservation efforts.